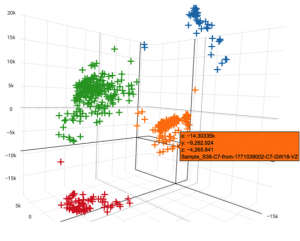
User Behavior Prediction in Programmatic RTB Advertising
Programmatic
Aug 17 2017
One of the biggest challenges in digital marketing is figuring out how to identify a potential customer online. Retargeting enables an advertiser to show ads to users who’ve been to their website already, but what about new customer acquisition? Should an advertiser leverage their existing customer data, 2nd party data, 3rd party data, or even just marketing intuition? Data science simplifies the answer by providing tools to make high impact, data driven decisions.
The following two strategies achieve the same goal of predicting whether a user will complete a conversion on the advertiser’s website (ecommerce purchase, contact us form fill, phone call, etc). The first is easily accessible and quick to implement, while the second requires significant investment to reap even greater rewards. This information is used to inform your campaign: who you should target, where budget should be spent, the potential return on ad spend, and ultimately, your bids. The outputs of these strategies are used to either manually tune campaigns or build an automated bidding algorithm.
Look-a-like Modeling
Google, Facebook, LinkedIn and countless other adtech players have quickly realized the value in look-a-like models. The idea is simple: upload a list of your highest value customers and the machine will first build a profile of those customers (like a persona or target market) and then rate users across the internet on how similar they are to that profile. It predicts a user’s behavior based on how similar they are to existing customers. If a user is very similar, then they are likely to also be a high value customer for your business. If a user is dissimilar, they won’t be valuable and are excluded from the targeting.
Facebook’s look-a-like models are the best in the industry due to their collection of private data on billions of individuals. The ecommerce brand, Modanisa, achieved a 4x ROAS and a 30% higher conversion rate compared to their previous campaign after implementing a look-a-like model.
Look-a-like models are excellent at finding more customers who are similar to your existing ones. But this method is flawed – not all your customers look the same. Other statistical models can look at how your past campaigns (or other third party data) have performed and identify groups of users who are highly likely to convert, even if they are dissimilar to most of your current customers. These strategies are significantly more costly to implement, but for good reason.
Predicting User Behavior
Rather than looking at current customers, other statistical models look at the landscape when the ad was shown and identify what factors meaningfully led to a conversion. In the example below each data point represents a single impression and its height represents the predicted conversion rate. Changing one factor, like the browser, changes the predicted outcome.
One tried and true method to predict conversion rates is called K-means clustering. It takes all these factors and groups individual impressions into clusters. The cluster with the highest predicted conversion rate (blue) is the target market. The users who are least likely to convert (red) are clustered at the bottom of the graph. Two clusters with medium potential to convert (green, orange) that should be explored are shown in the middle.
For example, the graph above was generated from a database of purchases for an ecommerce store that sells dried fruit. The blue cluster, their known target market, represents individual consumers who buy dried fruits for snacks. The green cluster represents a secondary market the brand is already aware of – small convenience stores who buy products in bulk, but much less often than consumers. The orange cluster, who the brand hadn’t previously known existed, represents restaurant owners who buy the dried fruit to cook with. The brand was unaware that this market existed and can now explore potential opportunities within it. This cluster wouldn’t have been identified by a look-a-like model because they are entirely different than most existing customers.
In a comparable case to K-means clustering, a group of researchers implemented a CTR prediction model with logistic regression and decision trees that outperformed traditional methods by over 3% and with significant impact to the overall system performance.
Looking Forward
Both of these methods are great for acquiring new customers. Look-a-like models can find customers similar to your existing ones and are easily accessible. Other methods like K-means clustering require an in-house data scientist to implement. While more difficult and expensive, these other methods are capable of finding customers that would otherwise never be identified.
With the growing use of machine learning, finding new customers is becoming easier and easier. In the coming years advanced statistical models that outperform look-a-like models will become accessible via 3rd party tools and eventually integrated into platforms like Facebook and Google. Once customers are identified, a bidding algorithm can put ads in front of these users for the lowest price.